Data closed loop research: in the stage of Autonomous Driving 3.0, work hard on end-to-end development to control data.
At present, autonomous driving has entered the stage 3.0. Differing from the software-driven stage 2.0 based on artificial rules, in the stage 3.0, the iteration of autonomous driving functions is driven by big data and foundation models; the perception mode is that multimodal sensors jointly output the results, while the information fusion mode evolves from post-fusion to in-fusion and pre-fusion.
As concerns data capability building, companies have shifted their focus to the efficiency and cost of the data closed loop, and can cope with more Corner Cases (extreme cases, e.g., traffic accidents, severe weathers or complex road conditions) on the premise of data compliance and data security, so as to expedite the development of user experience from "fatigue relief" to "scenario-based comfortable experience". Wherein, OEMs concentrate their efforts on improving application of autonomous driving in such scenario as city areas, urban overpasses and highways.
In highway scenarios, users can securely enable autonomous driving functions. The autonomous driving system provides a guide for accurate and comfortable on-ramp and off-ramp, keeps vehicles centering in lanes, allows vehicles to come around sharp corners at high speeds, balances follow and stop on congested road sections, intelligently selects the best lane, horizontally avoids and overtakes slow vehicles in lanes, and intelligently recognizes and avoids accidents and slow vehicles. For example, Pony.ai's NOA solution (see the figure below) makes a flexible response to highway and urban traffic scenarios, and performs well in such scenarios as automatic lane change, on/off-ramp, cruise control, lane centering, and obstacle avoidance.
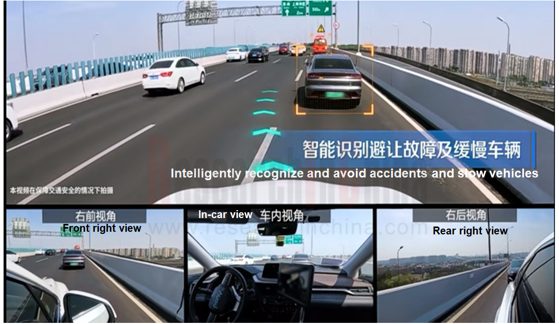
In urban scenarios, city NOA functions like NIO NOP+, Huawei NCA, and Xpeng XNGP have come into service, with the improving scenario generalization capabilities for vehicle functions. For example, Huawei’s intelligent driving system ADS2.0 mounted on Avatr allows the car to successfully pass through between the two trucks on a narrow urban street (see the figure below), and rivals or even outperforms humans in making decisions.
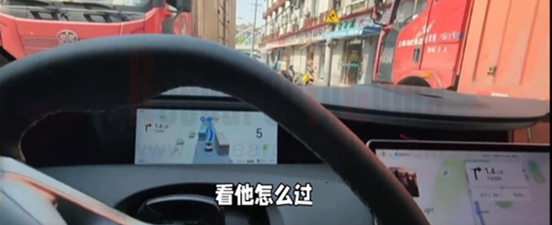
In short, the autonomous driving system performs ever better in automatic lane change, obstacle avoidance and other scenarios, and lets drivers take control of vehicles less frequently. Behind the vehicle intelligence competition is the efficient flow of data in the vehicle-cloud closed loop. Examples include Baidu AI Cloud’s autonomous driving data closed loop solution that provides full-cycle autonomous driving data operation services and an autonomous driving tool chain platform to solve problems in the process of data collection, processing, and use.
1. The stage of data-driven Autonomous Driving 3.0 starts, and companies double down on building or improving all the links of their data closed loop system.
The essence of the Autonomous Driving 3.0 is to be driven by data for ever higher data mining efficiency and date utilization. In this stage, the scale of vehicle test data can cover more than 100 million kilometers, which poses challenges to the collection, annotation and other links in the data closed loop. In this regard, automakers raise the efficiency of data processing by way of improving the flexible collection logic of the shadow mode and simulating zero prototypes to gear up for algorithm iteration, model training and deployment.
Let’s look at automakers’ measures to improve efficiency from the following key links of data closed loop, i.e., data collection, data annotation, and simulation test.
Data collection
At present, data can be collected by road collection vehicles, production vehicles, cloud simulation, and vehicle owner contribution. Wherein, the shadow mode works relatively more efficiently when collecting dynamic/static data inside and outside the vehicle. The algorithm trigger logic set by automakers is more flexible and precise. For example, Xpeng sets more than 300 trigger signals on vehicles with the ability to collect data, and the system can judge which corner case is useful at present and then uploads it. SAIC Rising Auto sets up multiple Triggered Events in cars to collect and return multimodal data after triggering these conditions, having enabled backhaul of nearly 12 million clips of data just within three months. In addition, EXCEEDDATA’s data collection system uses the cloud low-level code tool vStudio and various operators, making it easy to build trigger algorithms. The complete vehicle-cloud cooperative solution allows for one-click distribution of trigger algorithms to vehicles, without needing a complicated OTA process, delivering high algorithm iteration efficiency. EXCEEDDATA’s shadow mode is evolving to the stage 2.0, helping to build a trigger scene library and enable human-computer comparison and AB model comparison, and the edge computing assists in mining unknown abnormal scenarios for far lower cost and much higher efficiency.
Data annotation
Data annotation is one of the most critical links in the autonomous driving data closed loop. At present, how to keep improving the efficient automatic annotation of collected multimodal high-value data is the focus of conventional annotation companies and data closed loop solution providers.
To better empower automakers, conventional annotation companies are developing their own automated data annotation platforms to improve the efficiency and quality of data annotation. Meanwhile, they have also begun to partner with various intelligent computing centers, and use foundation models for ever greater capabilities of annotation platforms and lower cost of annotation.
In DataOcean AI’s case, its self-developed autonomous driving annotation platform DOTS-AD can support multi-dimensional, all-round autonomous driving annotation tasks, with up to 8 times higher data annotation efficiency. Another example is the MindFlow SEED data service platform which drives the mass annotation of autonomous driving data via AI+RPA, increases the comprehensive labor efficiency by an average of 30% and lowers the data production cost by an average of 40%.
In terms of driving scene recognition capabilities, the Haomo DriveGPT-based 4D Clips driving scene recognition solution built by Haomo.ai, a data closed loop solution provider, can cut down the annotation cost of a single picture to 0.5 yuan, a tenth of the current industry average cost. Haomo.ai is opening up the image frame and 4D Clips automatic driving scene recognition service to the industry. At present, quite a few annotation companies have joined hands with Haomo.ai, including Datatang, DataOcean AI, Appen, Testin and Stardust AI. The win-win cooperation between companies is bound to slash the data use cost for the industry and improve data quality.
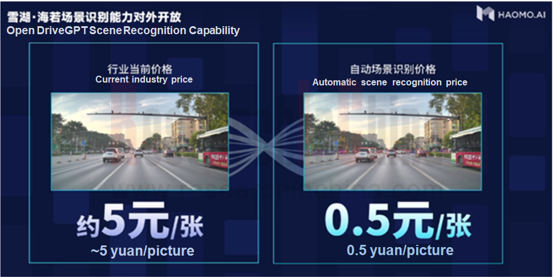
Simulation test
Cloud simulation platform construction is one of the tool chain capabilities of companies. The purchase and maintenance of simulation equipment is a cost pressure. Yet in the autonomous driving R&D process, compared with the approach using component prototypes to verify functions, virtual simulation needs fewer or zero prototypes. The higher cost performance makes simulation investment almost a must.
There are several simulation forms: MiL (model-in-the-loop), SiL (software-in-the-loop), HiL (hardware-in-the-loop), DiL (driver-in-the-loop), and ViL (vehicle-in-the-loop). Companies require a different simulation form in different stages of their R&D cycle. Currently many automakers are using simulation tools to verify functions and shorten the R&D cycle and the time-to-market of new models. For example, VI-grade’s products are used by BMW, Mercedes-Benz, Audi, Ford, Honda, Toyota, SAIC, FAW, GAC and NIO among others.
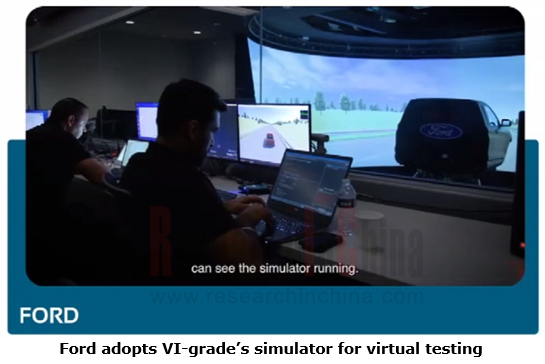
2. When constructing a data closed loop ecosystem, companies quicken their pace of building "digital intelligence/data base" capabilities.
Data, algorithm, and computing power are the three cornerstones of autonomous driving technology. The volume and quality of data determine the upper limit of algorithm capabilities, and computing power is the carrier of data processing. The integration of software and hardware means the "smoothness" of adaptability between algorithms and domain controller/chip. Currently just a few companies like Tesla in the industry have built a complete intelligent ecosystem of "data + algorithm + computing power", and take 100% control of data. To have control over data, OEMs and software algorithm companies are running after them.
Build intelligent computing centers
As the initial investment in supercomputing centers is relatively high, OEMs and Tier 1 suppliers generally budget over RMB100 million for building AI computing centers. In the case of Dojo, Tesla’s supercomputer platform that was put into use in July 2023, Tesla will invest more than USD1 billion in Dojo in 2024 to facilitate training of the supercomputer and neural networks.
Even so, automakers or technology providers with a long-term plan for autonomous driving are building their own supercomputing centers to hold stable computing resources and shorten the development cycle and the time-to-market of autonomous driving products. One example is "Fuyao", Xpeng’s autonomous driving AI intelligent computing center co-funded with Alibaba in August 2022. The center can speed up the model training of autonomous driving algorithms by 170 times, and has the scope to increase computing power by 10 to 100 times in the future.
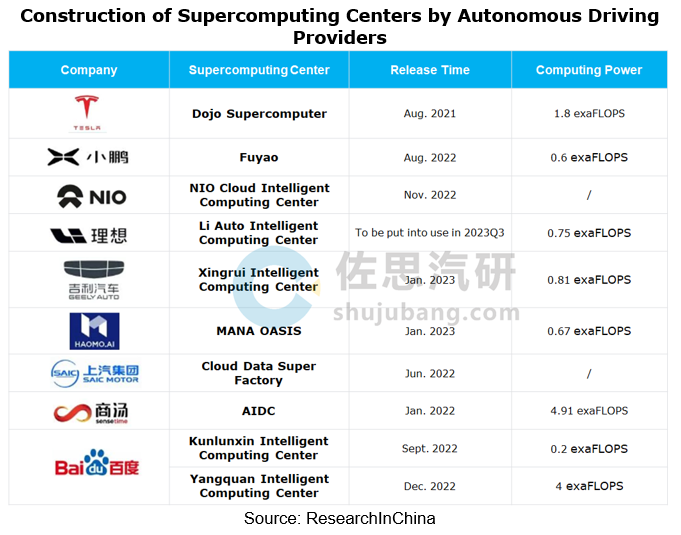
"Digital intelligence/data base" capability building
The full life cycle of intelligent vehicles needs to be driven by data, and it is the data-based vehicle-cloud full-link capability base that some autonomous driving solution providers work hard to build. For example, ExceedData’s vehicle-cloud integrated computing architecture combines with its vehicle high-performance time-series database to build a data base for intelligent vehicles, and redefines the cost and efficiency of vehicle data intelligence, enabling an 85% reduction in the total cost. The data base solution has been well accepted by first-tier automakers including FAW, SAIC, SAIC Z-ONE, Human Horizons, Dongfeng Voyah, BAIC and Geely, and has been mass-produced and designated for more than 10 vehicle models.
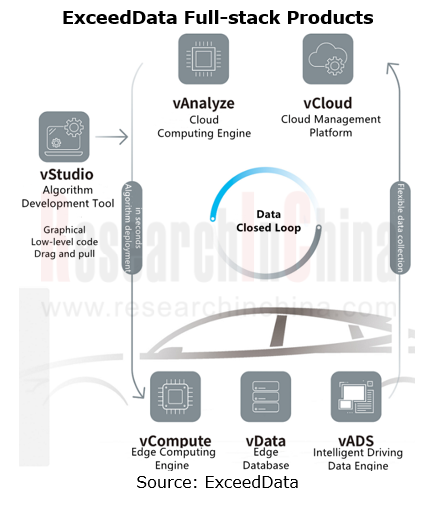
Freetech has the ability to develop, mass-produce and deliver software and hardware integrated platforms, and has built a data closed loop link of "large-scale data collection - data processing system - automated iteration". Freetech’s ODIN intelligent driving digital intelligence base supports large-scale mass production data closed loop system, and is composed of Freetech’s large-scale mass production data base and the computing power platform deployed at the National Super Computing Center. Freetech has built a complete data closed loop system that supports algorithm evolution, and can complete the iterative evolution of perception algorithms, and the closed-loop verification of planning and control algorithms. The ODIN digital intelligence base is composed of self-developed domain controller, sensor, autonomous driving algorithm, and data closed loop system as the four pillar systems, having cooperated with more than 40 auto brands on over 100 model projects.
3. BEV+Transformer enables end-to-end perception and decision integration.
The enhancement of autonomous driving capabilities is the result of the joint optimization of “more data + better algorithms + higher computing power”, and it is also the inevitable result of advancement of perception, decision, planning and control technologies.
A variety of complex scenarios, especially corner cases, require higher perception and decision capabilities of autonomous driving. BEV technology enhances the perception of autonomous driving systems by providing a holistic perspective. Transformer models can be used to extract features from multimodal data, such as LiDAR point clouds, images and radar data. The end-to-end training on these data allows Transformer to automatically learn the internal structure and interrelation of these data, so as to effectively recognize and locate obstacles in environments.
BEV+Transformer can build an end-to-end autonomous driving system to achieve high-precision perception, prediction and decision. In SenseTime’s case, based on a multimodal foundation model, it can enable data closed loops of prediction and decision. UniAD, SenseTime's perception-decision integrated end-to-end autonomous driving solution, increases the lane line prediction accuracy by 30%, and reduces the errors of motion displacement prediction by nearly 40% and the planning errors by nearly 30%. With regard to AI decision, SenseTime and Shanghai Artificial Intelligence Laboratory launched OpenDILab, a decision AI platform which can also be used in autonomous driving for planning and control.
In addition, JueFX Technology also has the "perception-decision-data" closed-loop capability, and its competitive edge lies in the integration and flywheel effect between algorithms and data. In terms of software, JueFX’s core technology path is the fusion of computing power, that is, fuse the spatial data related to the position and attitude of the vehicle, the static data of heavy or light maps, and the sensor perception data, compute them in real time, and apply them to the perception system, positioning system, path planning system or memory mapping system of autonomous driving.
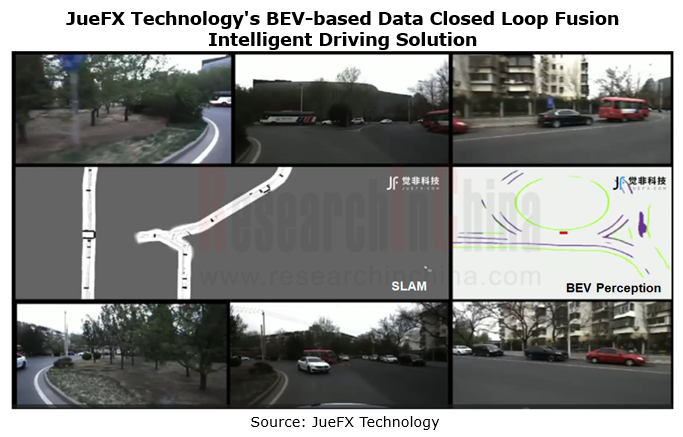
China Passenger Car Electronic Control Suspension Industry Research Report, 2025
Electronic control suspension research: air springs evolve from single chamber to dual chambers, CDC evolves from single valve to dual valves
ResearchInChina released "China Passenger Car Elect...
Automotive XR Industry Report, 2025
Automotive XR industry research: automotive XR application is still in its infancy, and some OEMs have already made forward-looking layout
The Automotive XR Industry Report, 2025, re...
Intelligent Driving Simulation and World Model Research Report, 2025
1. The world model brings innovation to intelligent driving simulation
In the advancement towards L3 and higher-level autonomous driving, the development of end-to-end technology has raised higher re...
Autonomous Driving Map (HD/LD/SD MAP, Online Reconstruction, Real-time Generative Map) Industry Report 2025
Research on Autonomous Driving Maps: Evolve from Recording the Past to Previewing the Future with "Real-time Generative Maps"
"Mapless NOA" has become the mainstream solution for autonomous driving s...
End-to-End Autonomous Driving Research Report, 2025
End-to-End Autonomous Driving Research: E2E Evolution towards the VLA Paradigm via Synergy of Reinforcement Learning and World Models??The essence of end-to-end autonomous driving lies in mimicking dr...
Research Report on OEMs and Tier1s’ Intelligent Cockpit Platforms (Hardware & Software) and Supply Chain Construction Strategies, 2025
Research on intelligent cockpit platforms: in the first year of mass production of L3 AI cockpits, the supply chain accelerates deployment of new products
An intelligent cockpit platform primarily r...
Automotive EMS and ECU Industry Report, 2025
Research on automotive EMS: Analysis on the incremental logic of more than 40 types of automotive ECUs and EMS market segments
In this report, we divide automotive ECUs into five major categories (in...
Automotive Intelligent Cockpit SoC Research Report, 2025
Cockpit SoC research: The localization rate exceeds 10%, and AI-oriented cockpit SoC will become the mainstream in the next 2-3 years
In the Chinese automotive intelligent cockpit SoC market, althoug...
Auto Shanghai 2025 Summary Report
The post-show summary report of 2025 Shanghai Auto Show, which mainly includes three parts: the exhibition introduction, OEM, and suppliers. Among them, OEM includes the introduction of models a...
Automotive Operating System and AIOS Integration Research Report, 2025
Research on automotive AI operating system (AIOS): from AI application and AI-driven to AI-native
Automotive Operating System and AIOS Integration Research Report, 2025, released by ResearchInChina, ...
Software-Defined Vehicles in 2025: OEM Software Development and Supply Chain Deployment Strategy Research Report
SDV Research: OEM software development and supply chain deployment strategies from 48 dimensions
The overall framework of software-defined vehicles: (1) Application software layer: cockpit software, ...
Research Report on Automotive Memory Chip Industry and Its Impact on Foundation Models, 2025
Research on automotive memory chips: driven by foundation models, performance requirements and costs of automotive memory chips are greatly improved.
From 2D+CNN small models to BEV+Transformer found...
48V Low-voltage Power Distribution Network (PDN) Architecture and Supply Chain Panorama Research Report, 2025
For a long time, the 48V low-voltage PDN architecture has been dominated by 48V mild hybrids. The electrical topology of 48V mild hybrids is relatively outdated, and Chinese OEMs have not given it suf...
Research Report on Overseas Cockpit Configuration and Supply Chain of Key Models, 2025
Overseas Cockpit Research: Tariffs stir up the global automotive market, and intelligent cockpits promote automobile exports
ResearchInChina has released the Research Report on Overseas Cockpit Co...
Automotive Display, Center Console and Cluster Industry Report, 2025
In addition to cockpit interaction, automotive display is another important carrier of the intelligent cockpit. In recent years, the intelligence level of cockpits has continued to improve, and automo...
Vehicle Functional Safety and Safety Of The Intended Functionality (SOTIF) Research Report, 2025
Functional safety research: under the "equal rights for intelligent driving", safety of the intended functionality (SOTIF) design is crucial
As Chinese new energy vehicle manufacturers propose "Equal...
Chinese OEMs’ AI-Defined Vehicle Strategy Research Report, 2025
AI-Defined Vehicle Report: How AI Reshapes Vehicle Intelligence?
Chinese OEMs’ AI-Defined Vehicle Strategy Research Report, 2025, released by ResearchInChina, studies, analyzes, and summarizes the c...
Automotive Digital Key (UWB, NearLink, and BLE 6.0) Industry Trend Report, 2025
Digital key research: which will dominate digital keys, growing UWB, emerging NearLink or promising Bluetooth 6.0?ResearchInChina has analyzed and predicted the digital key market, communication techn...