Research on autonomous driving SoC: driving-parking integration boosts the industry, and computing in memory (CIM) and chiplet bring technological disruption.
“Autonomous Driving SoC Research Report, 2023” released by ResearchInChina highlights mainstream automakers’ autonomous driving SoC and system deployment strategies, and 9 overseas and 10 Chinese autonomous driving SoC vendors, and discusses the following key issues:
Analysis and outlook for autonomous driving SoC and system deployment strategies of OEMs;
Application and configuration strategy of autonomous driving SoC in driving-parking integration;
Application trends of autonomous driving SoC in cockpit-driving integration;
Recommended "Turnkey" SoC solutions for autonomous driving;
Autonomous driving SoC product selection and cost analysis;
Is it feasible for OEMs to independently make chips (autonomous driving SoC)?
Application of chiplet in autonomous driving SoC;
Application of computing in memory (CIM) in autonomous driving SoC.
In driving-parking integration market, single-SoC and multi-SoC solutions have their own target customers.
At this stage, Mobileye still rules the roost in the entry level L2 (intelligent front view all-in-one). In the short term, new products like TI TDA4L (5TOPS) pose a challenge to Mobileye in L2. For L2+ driving and driving-parking integration, most automakers currently adopt multi-SoC solutions. Examples include Tesla’s "dual FSD", “triple Horizon J3” on Roewe RX5, "Horizon J3 + TDA4"on Boyue L and Lynk & Co 09, and "dual ORIN" on NIO ET7, IM L7 and Xpeng G9/P7i among others.
According to the production deployment plans of OEMs and Tier 1 suppliers, for lightweight (cost-effective) driving-parking integration, the fusion of driving and parking domains complicates the embedded system design, and poses higher requirements for algorithm model, chip computing power calling (time division multiplexing), computational efficiency of SoC, and costs of SoC and domain control materials.
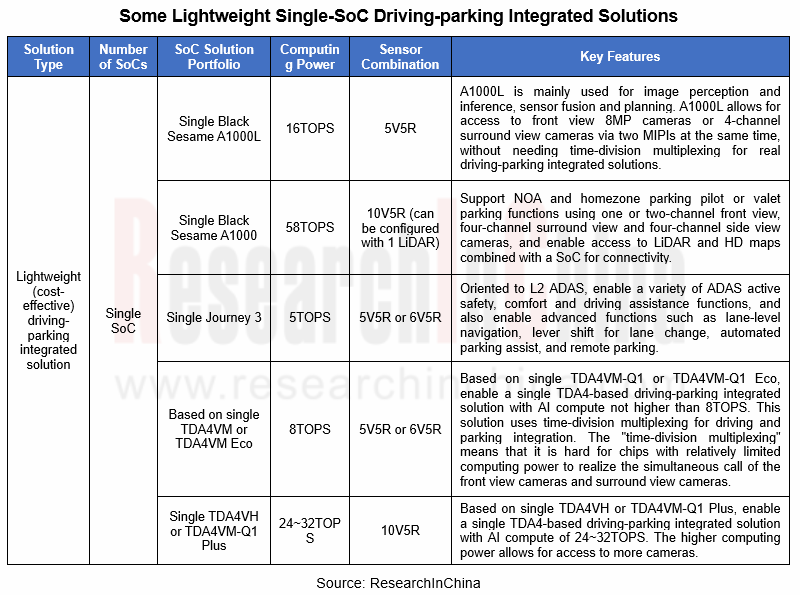
Cost-effective single-SoC solutions: for passenger cars valued at RMB100,000-200,000, the mass production and deployment of the solutions will peak in 2023. The single-SoC driving-parking integrated solutions generally use Horizon J3/J5, TI TDA4VM/TDA4VH/TDA4VM-Q1 Plus, and Black Sesame A1000/A1000L chips. With cost advantages, they can further lower the BOM cost (bill of materials) of entire domain controllers. For example, based on single A1000 SoC and supporting the 10V (camera) NOA function, Black Sesame’s driving-parking integrated solution can enable the reduction of the BOM cost of domain controllers to less than RMB3,000, and supports 50-100T physical computing power.
Cost-effective multi-SoC solutions: oriented to passenger cars valued at RMB150,000-250,000, overlapping with those carrying single-SoC solutions, the solutions contain dual TDA4, Horizon J2/J3+TDA4, dual Horizon J3, dual EQ5H, dual Horizon J3+NXP S32G, and triple Horizon J3. Multi-SoC solutions remain superior in safety redundancy and reserve space for OTA updates.
High-level driving-parking integration needs access to more cameras with higher resolution, as well as 4D radars and LiDAR. The BEV+Transformer neural network model is larger and more complex, and may even need to support local algorithm training, so it requires high enough computing power, CPU compute up to at least 150KDMIPS, and AI compute up to least 100TOPS.
High-level driving-parking integration targets high-end new energy vehicles priced at not lower than RMB250,000, with low price sensitivity but higher requirements for power consumption and efficiency of AI chips. In particular, high-compute chips have an impact on the endurance range of new energy vehicles, so that chip vendors have to introduce ever more advanced processes and more energy-efficient chip products.
High-end single SoC solutions: single Horizon J5 and single Black Sesame A1000/A1000 pro solutions gain popularity, and support the application and deployment of 1-2L+11V+5R and leading intelligent driving algorithm models like BEV. In the next stage, single Qualcomm Snapdragon Ride, single Ambarella CV3-AD, and single ORIN chips may also be used by some OEMs as main solutions.
?High-end multi-SoC solutions: dual Nvidia Orin-X and dual FSD are still the mainstream solutions for most of mid- and high-end new energy vehicle models, including the full range of Tesla models, Li Auto L9, Xpeng G9/P7i, IM L7 and Lotus. NIO ET7/ET5 even uses four Orin-X SoCs, two for daily driving computation, and the other two for algorithm training and backup redundancy.
Autonomous driving is facing the contradiction between high computing power and low power consumption, and CIM AI chips may become the ultimate solution.
The popularity of ChatGPT indicates the development directions of autonomous driving: foundation models and high computing power. For large neural network models such as Transformer, the computation will multiply by 750 times every two years on average; for video, natural language processing and speech models, the computation will increase by 15 times every two years on average. It is conceivable that Moore's Law will cease to apply, and the "storage wall" and "power consumption wall" will become the key constraints on the development of AI chips.
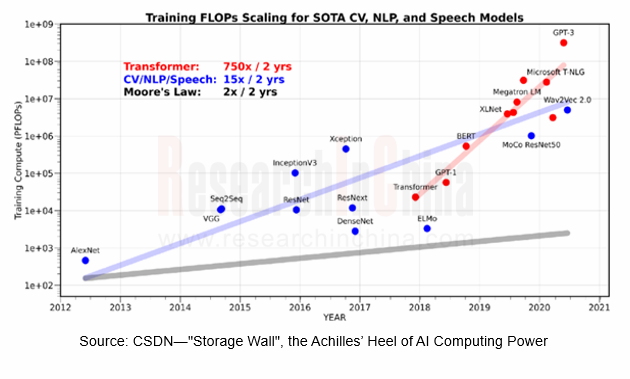
At present, most of conventional computing architectures are von Neumann architecture with high flexibility. Yet the problems faced by AI chips are computing power bottleneck and large data transfer, which bring high power consumption.
The computing in memory (CIM) technology is expected to be a solution to the contradiction between high computing power and low power consumption. Computing in memory (CIM) refers to data operation in memory to avoid the "storage wall" and "power consumption wall" caused by data transfer and enable far higher parallelism and energy efficiency of data.
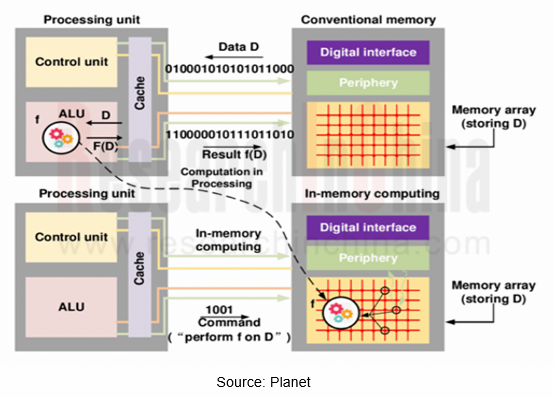
In the automotive field, highly autonomous vehicles will become a running supercomputing center in a sense, with increasing computing power, up to more than 1000TOPS. Cloud computing has sufficient power and can cool down via cooling system, while vehicle edge computing is powered by a battery, facing problems of liquid cooling and cost at the same time.
CIM AI chips will be a new technology path option for automakers.
In the field of autonomous driving SoC, Houmo.ai is the first autonomous driving CIM AI chip vendor in China. In 2022, it successfully lightened the industry's first high-compute CIM AI chip on which the intelligent driving algorithm model runs smoothly. This verification sample uses a 22nm process and boasts computing power of 20TOPS, which can be expanded to 200TOPS. Noticeably the energy efficiency ratio of its computing unit is as high as 20TOPS/W. It is known that Houmo.ai will introduce a production-ready intelligent driving CIM chip soon, and we will share its performance in the report.
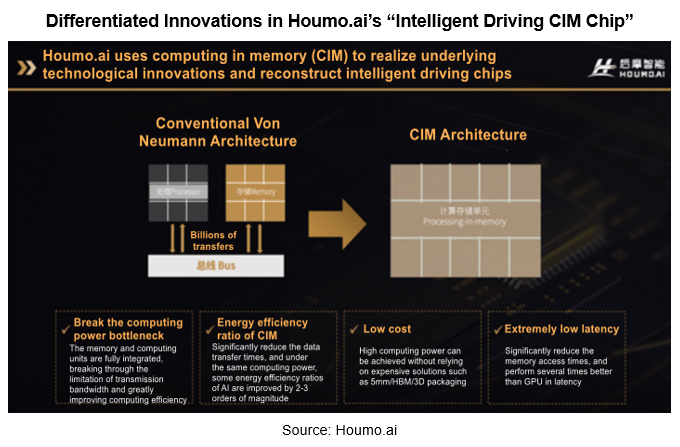
In the future, as with power batteries, chips will become an investment hotspot for large OEMs.
That OEMs make chips is an extremely controversial issue. In the industry, it is a popular belief that on one hand, OEMs cannot rival specialist IC design companies in development speed, efficiency, and product performance; on the other hand, only when the shipment of a single chip reaches at least one million units can its development cost can be continuously diluted to make it cost-effective.
But in fact, chips have played an absolutely dominant part in intelligent connected new energy vehicles in performance, cost, and supply chain safety. Compared with the typical fuel-powered vehicle that needs 700-800 chips, a new energy vehicle needs 1,500-2,000 units, and a highly autonomous new energy vehicle even needs as many as 3,000 units, some of which are highly valued, high-cost chips that may be in short supply and even out of stock.
It is obvious that large OEMs do not want to be bound by some chip vendor, and they even have already begun to manufacture chips independently. In Geely’s case, the automaker has spawned 7nm cockpit SoCs and installed them in vehicles, and has also accomplished IGBT tape-out. The autonomous driving SoC AD1000, jointly developed by ECARX and SiEngine, is expected to be taped out in March 2024 at the earliest.
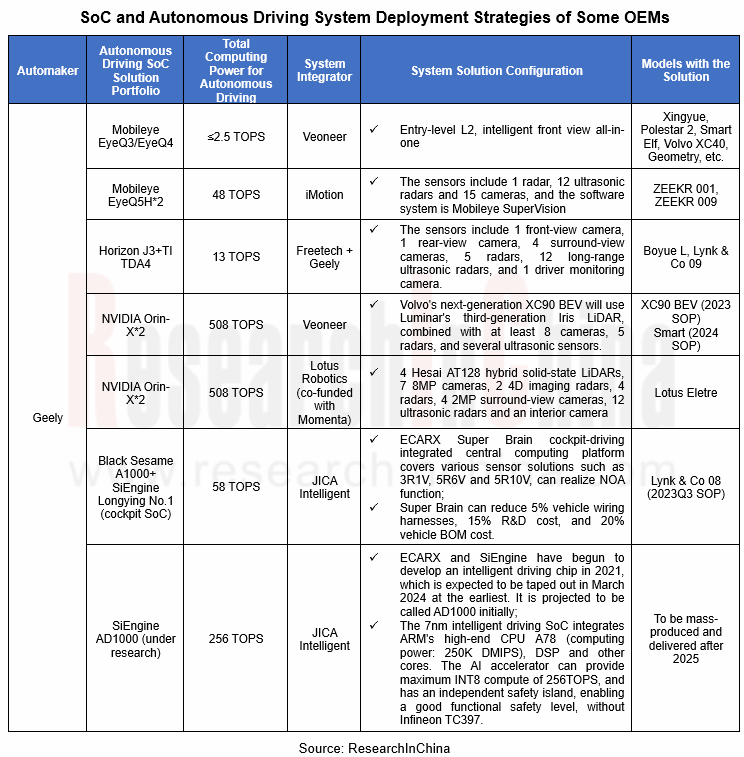
We predict that as with power batteries, chips will become an investment hotspot for large OEMs to strengthen their underlying basic capabilities. In 2022, Samsung announced that it will make chips for Waymo, Google's self-driving division; GM Cruise also announced independent development of autonomous driving chips; Volkswagen announced that it will establish a joint venture with Horizon Robotics, a Chinese autonomous driving SoC vendor.
At the China EV100 Forum 2022, Horizon Robotics opened the IP license of BPU (Brain Processing Unit), its high-performance autonomous driving processor architecture on the basis of its business model of "chip + algorithm + tool chain + development platform", in a bid to meet the needs of some automakers with great ability to develop independently, thereby improving their differentiated competitive edges and accelerating their pace of R&D and innovation. As an IP provider that supports automakers to self-develop computing solutions, Horizon Robotics has confirmed a BPU IP licensing model partner and is developing another automaker partner.
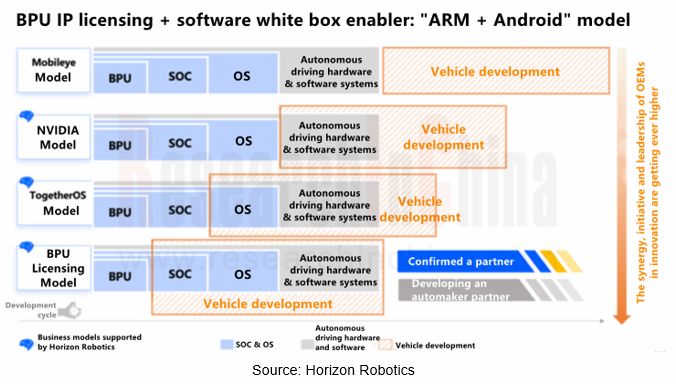
The technical barriers for chip fabrication are not particularly high. The primary threshold is enough capital and order intake. The chip industry now adopts the block-building model, namely, purchasing IPs to build chips including CPU, GPU, NPU, storage, NoC/bus, ISP and video codec. In the future, as chiplet ecosystems and processes get improved, the threshold for independent development of autonomous driving SoCs will be much lower for automakers just need to buy dies (IP chip) directly and then package them, with no need to buy IPs.
In the case of Tesla HW 3.0, the architecture design is based on Samsung Exynos-IP; the CPU/GPU/ISP design uses ARM’s IP; the network-on-chip (NoC) uses Arteris’ IP. Tesla only self-develops neural network accelerator (NNA) IP, and the foundry is Samsung.
Tesla deepens its cooperation with Broadcom on HW 4.0 development. To improve AI computing power, the easiest and most effective way is to stack up MAC units and SRAM. For AI operations, the main bottleneck is storage. The disadvantage is that SRAM occupies a large space of chips, and the chip area is however proportional to the cost. Moreover, it is difficult to increase the density and reduce the area of SRAM using advanced processes.
Therefore the area of Tesla's first-generation bare chip FSD HW 3.0 is 260 square millimeters, and the area of the second-generation bare chip FSD HW 4.0 is expected to be up to 300 square millimeters, with the total cost estimated to increase by at least 40-50%. By our estimate, the cost of HW3.0 chips has dropped to USD90-100, and HW 4.0 should cost USD150-200, but even so, Tesla’s self-developed chips are far more cost-effective than the bought-in.
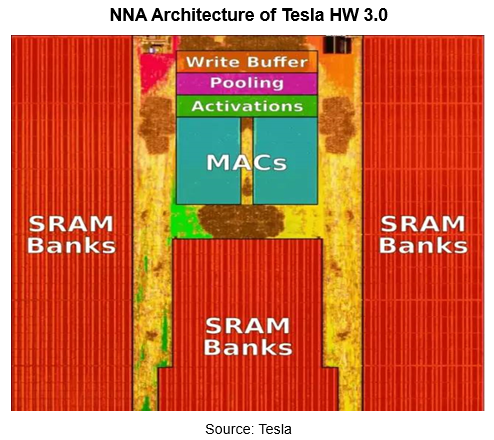
In the long run, OEMs with millions of sales are feasible to make chips on their own.
Auto Shanghai 2025 Summary Report
The post-show summary report of 2025 Shanghai Auto Show, which mainly includes three parts: the exhibition introduction, OEM, and suppliers. Among them, OEM includes the introduction of models a...
Automotive Operating System and AIOS Integration Research Report, 2025
Research on automotive AI operating system (AIOS): from AI application and AI-driven to AI-native
Automotive Operating System and AIOS Integration Research Report, 2025, released by ResearchInChina, ...
Software-Defined Vehicles in 2025: OEM Software Development and Supply Chain Deployment Strategy Research Report
SDV Research: OEM software development and supply chain deployment strategies from 48 dimensions
The overall framework of software-defined vehicles: (1) Application software layer: cockpit software, ...
Research Report on Automotive Memory Chip Industry and Its Impact on Foundation Models, 2025
Research on automotive memory chips: driven by foundation models, performance requirements and costs of automotive memory chips are greatly improved.
From 2D+CNN small models to BEV+Transformer found...
48V Low-voltage Power Distribution Network (PDN) Architecture and Supply Chain Panorama Research Report, 2025
For a long time, the 48V low-voltage PDN architecture has been dominated by 48V mild hybrids. The electrical topology of 48V mild hybrids is relatively outdated, and Chinese OEMs have not given it suf...
Research Report on Overseas Cockpit Configuration and Supply Chain of Key Models, 2025
Overseas Cockpit Research: Tariffs stir up the global automotive market, and intelligent cockpits promote automobile exports
ResearchInChina has released the Research Report on Overseas Cockpit Co...
Automotive Display, Center Console and Cluster Industry Report, 2025
In addition to cockpit interaction, automotive display is another important carrier of the intelligent cockpit. In recent years, the intelligence level of cockpits has continued to improve, and automo...
Vehicle Functional Safety and Safety Of The Intended Functionality (SOTIF) Research Report, 2025
Functional safety research: under the "equal rights for intelligent driving", safety of the intended functionality (SOTIF) design is crucial
As Chinese new energy vehicle manufacturers propose "Equal...
Chinese OEMs’ AI-Defined Vehicle Strategy Research Report, 2025
AI-Defined Vehicle Report: How AI Reshapes Vehicle Intelligence?
Chinese OEMs’ AI-Defined Vehicle Strategy Research Report, 2025, released by ResearchInChina, studies, analyzes, and summarizes the c...
Automotive Digital Key (UWB, NearLink, and BLE 6.0) Industry Trend Report, 2025
Digital key research: which will dominate digital keys, growing UWB, emerging NearLink or promising Bluetooth 6.0?ResearchInChina has analyzed and predicted the digital key market, communication techn...
Integrated Battery (CTP, CTB, CTC, and CTV) and Battery Innovation Technology Report, 2025
Power battery research: 17 vehicle models use integrated batteries, and 34 battery innovation technologies are released
ResearchInChina released Integrated Battery (CTP, CTB, CTC, and CTV)and Battery...
AI/AR Glasses Industry Research Report, 2025
ResearchInChina released the " AI/AR Glasses Industry Research Report, 2025", which deeply explores the field of AI smart glasses, sorts out product R&D and ecological layout of leading domestic a...
Global and China Passenger Car T-Box Market Report 2025
T-Box Research: T-Box will achieve functional upgrades given the demand from CVIS and end-to-end autonomous driving
ResearchInChina released the "Global and China Passenger Car T-Box Market Report 20...
Automotive Microcontroller Unit (MCU) Industry Report, 2025
Research on automotive MCUs: the independent, controllable supply chain for automotive MCUs is rapidly maturing
Mid-to-high-end MCUs for intelligent vehicle control are a key focus of domestic produc...
Automotive LiDAR Industry Report, 2024-2025
In early 2025, BYD's "Eye of God" Intelligent Driving and Changan Automobile's Tianshu Intelligent Driving sparked a wave of mass intelligent driving, making the democratization of intelligent driving...
Software-Defined Vehicles in 2025: SOA and Middleware Industry Research Report
Research on automotive SOA and middleware: Development towards global SOA, cross-domain communication middleware, AI middleware, etc.
With the implementation of centrally integrated EEAs, OEM softwar...
Global and Chinese OEMs’ Modular and Common Technology Platform Research Report, 2025
Modular platforms and common technology platforms of OEMs are at the core of current technological innovation in automotive industry, aiming to enhance R&D efficiency, reduce costs, and accelerate...
Research Report on the Application of AI in Automotive Cockpits, 2025
Cockpit AI Application Research: From "Usable" to "User-Friendly," from "Deep Interaction" to "Self-Evolution"
From the early 2000s, when voice recognition and facial monitoring functions were first ...